Where you are reading Pi (3,14) in
the following paper from 1992, you should replace Pi by 2 Phi
(3,236) -
see: Weiss, Harald and Volkmar Weiss: The
golden mean as clock cycle of brain waves. Chaos, Solitons and
Fractals 18 (2003) 643-652 - Elsevier Author Gateway, online
version -
or v-weiss/chaos.html
-------------------------------------------------------------------------------------------------
published in: Biological
Cybernetics 68 (1992)165-172
The Relationship between Short-Term
Memory Capacity
and EEG Power Spectral
Density
Volkmar
Weiss
Abstract. Multiplying memory span by mental speed, we
obtain the information entropy of short-term memory capacity, which
is rate-limiting for cognitive functions and corresponds with EEG
power spectral density. The number of EEG harmonics (n = 1, 2, ... ,
9) is identical with memory span, and the eigenvalues of the EEG
impulse response are represented by the zero-crossings up to the
convolved fundamental, the P300. In analogy to quantum mechanics the
brain seems to be an ideal detector simply measuring the energy of
wave formes. No matter what the stimulus is and how the brain
behaves, the metric of signal and memory can always be understood as
a superposition of np states of
different energy and their eigenvalues.
1
Introduction During the last decade a number of papers and
books have been published, claiming in a more or less speculative
manner (for the current state of this art compare Penrose 1990) a
link between quantum mechanics and thought. Koenderink and Van Doorn
(1990) even found a "miraculous coincidence" between their partial
differential equations of receptive fields of vision and the
harmonic oscillator of quantum mechanics. And Vichnevetsky
discovered (1988) by simulation experiments "unexpectedly that wave
propagation in computational fluid dynamics and the motion of
particles in quantum mechanics share similar mathematics". However,
all these authors are seemingly unaware of a growing body of
empirical evidence, interpreted in the following and supporting
their arguments.
Already in 1966, Kac, had put forward the
question: Can one hear the shape of a drum? In order to find an
answer, Kac asks for the energy in the frequency interval df. To
this end, he calculates the number of harmonics which lie between
the frequencies f und df and multiplies this number by the energy
which belongs to the frequency f, and which according to the theory
of quantum mechanics is the same for all frequencies. By solving the
eigenvalue problem of the wave equation, Kac is able to state that
one can not only hear the area of a reflecting surface, its volume
and circumference, but also - and this seems to be the most exciting
result in view of modern network theories of brain machinery - the
connectivity of paths of an irregular shaped network. If the brain
waves had the possibility to measure and hence to "know" the
eigenvalues of a spatially distributed information amount, they
would have nearly perfect access to information and - in terms of
communication theory - perform nearly perfect bandlimited
processing. As we know, the eigenvalues are proportional to the
squares (i.e. variances) of resonant frequencies (Fogleman
1987).
The question whether brain waves reflect underlying
information processing is as old as EEG research itself. Such
relationships between well-confirmed psychometric and
psychophysiological empirical facts (compare also Eysenck 1986 and
1987) and EEG spectral density, and why, are the very problem.
Recently, Resnikoff (1989) has published a textbook which contains
the background of interdisciplinary knowledge, indispensible for
progress in a field of such sophistication.
2 Empirical Results
of NeoPiagetian Cognitive Psychology
Ever since
short-term memory became the object of scientific study,
psychologists have recognised that it possesses a quantitative
dimension in terms of the maximum number of items to which a person
can attend at one time. Attempts to measure this span of attention
revealed that it was indeed limited, but that those limits were
fluid and depended on a variety of factors. However, the discovery
of a constant in science, even a "fuzzy" one, is an important event.
It now seems almost universally accepted that short-term memory span
has a capacity limit of seven plus or minus two (Miller 1956). The
possibility that such quantitative limits on attention span might be
related to qualitative differences in thought and reasoning was
recognised by Piaget (see 1971) in his earliest research reports.
Beginning with Pascual-Leone (1970), the prediction of children`s
reasoning from estimates of their memory span has been a major goal
of neoPiagetian theories of cognitive development.
In the
pioneering research by Pascual-Leone (1970) the maximum of memory
span became the mental energy operator M. Maximum mental power (= M)
is characterised as the maximum absolute number of different schemes
(including overlearned complex subroutines and strategies) that can
be activated in a single mental act. Pascual-Leone (1970; p. 304)
understands memory span as the maximum of discrete and equal energy
units (i.e. quanta) which every subject has at his disposal.
"Assuming that M operates upon the units or behavioural segments
available in the subject`s repertoire, as the repertoire changes
with learning so will the level of performance even if the subject`s
M value remains constant. A distinction can and should be made
between the subject`s maximum capacity or structural M (Ms) and his
functional M (Mf) or amount of Ms space actually used by him at any
particular moment of his cognitive activity. It seems reasonable to
assume that the value taken by Mf oscillates between zero and Ms.
This functional Mf would constitute a discrete random variable which
can be influenced by a multiplicity of factors, from the degree of
motivational arousal and the degree of fatigue to some
individual-differences variables."
In the first step of
Pascual-Leone`s experimental procedure all subjects learned a small
repertoire of stimulus-response units. The number of units to be
learned varied across age groups as a function of the predicted
maximum M operator size for the group. That it, 5-year-olds learned
5 units, 7-year-olds 6 units, 9-year-olds 7 units, 11-year-olds 8
units. The stimuli forming the repertoire were easily discriminable
simple visual cues such as: square, red, dot-inside-the-figure,
etc.. The corresponding responses were overlearned motor behaviours
such as: raise-the-hand, hit-the-basket, clap-hands, etc.. The
universe of simple stimulus-response units used by Pascual-Leone in
the whole series of his experiments is presented in Table
1.
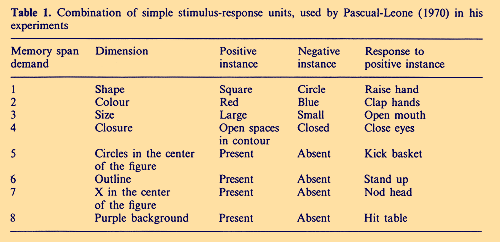
The experimental setting and instructions were as follows:
Different sets of cards constituted the stimulus material. Every
pair of cards illustrated a simple stimulus-response unit of a given
level (see Table 1). Experimenter and subject set facing each other
across a table. The task was introduced as a spy game. Experimenter
would teach subject a code and when he knew it well, experimenter
would send him some secret messages. The whole series was repeated
until the subject's motor responses were without error. When the
subject had learned his repertoire the second step was introduced. A
new randomly ordered set of cards was presented, exhibiting compound
stimuli of all possible combinations previously learned by the
subject. The subject` s task was to respond to any recognised
stimulus. Cards were presented manually and the exposure time was
fixed at 5 sec but the subject had free time for
responding.
Pascual-Leone`s application (1970; p. 318) of
Bose-Einstein statistics to his experimental data seems to be
especially remarkable. Because access to chunks in working memory is
random, the available energy quanta are not distinguishable. The
probability distribution of the random variable x (i.e. the number
of different responses produced by the subject) can be calculated on
the basis of the combinatorics of the total number c of cues in the
stimulus compound (i.e. the span demand of the task; compare Table
1) and the available energy quanta n. By applying the Bose-Einstein
occupancy model of combinatorics

|
(1) | to his learning
experiments with children of different age, Pascual-Leone obtained a
very good agreement between empirical probabilities Pe (x) and
Bose-Einstein predicted theoretical probabilities Pt (x) (see Table
2)
Table 2. Learning
experiments by Pascual-Leone (1970, Table6): Bose-Einstein
predicted theoretical (Pt) and empirical (Pe) probabilities of
compound responses in the sample of 11-year-olds (n=14;
experimental observations=1297; mean IQ=119, ranging from 106
to 131; mean age=11.8 years). |
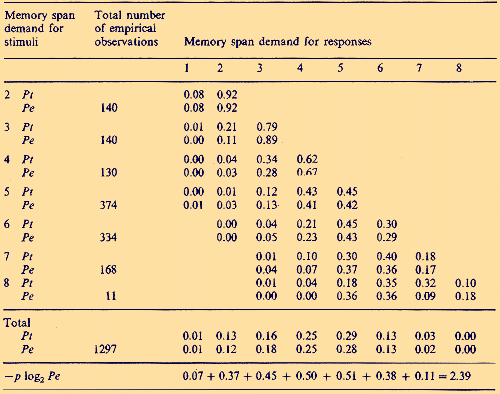 | As it seems, the deeper
meaning of this astounding agreement between a prediction based on a
formula of quantum statistics and an outcome of a psychological
experiment has never been discussed seriously. But it should. Now we
try to extend Pascual-Leone`s theory and results: We have added the
last row in Table 2, showing the corresponding -p log2 Pe (x). The
information entropy H of a system of indistinguishable particles
distributed over occupation number n equals (Yu 1976).

|
(2) | The sum of
logarithms to the base 2 of the probabilities that the state n is
occupied is 2.39 (see Table 2, last row). For 11-year-olds there are
8 possible quantum states (i.e. the maximum of memory span equals
the maximum of such states), hence 8 + 7 + 6 + 5 + 4 + 3 + 2 + 1 =
36. By multiplying 36 with 2.4 we get for this sample of subjects
a mean information entropy H of 86.4 bits. A mean IQ of 119 for 11.8
year-olds corresponds in performance to an adult IQ of 102 for about
40-year-olds. In tables of IQ test results edited by Lehrl et al.
(1991) and based on concepts of information theory, we read for this
age and IQ 102 a short-term memory storage capacity of 84 bits.
Thus, two completely different empirical approaches, one of
cognitive psychology the other of differential psychology, with
seemingly completely differing theoretical starting points lead on
the absolute scale of information entropy to practically the same
result. For Pascual-Leone`s data the latter result was even obtained
after applying quantum mechanics twice in series, for calculating
Bose-Einstein statistics (by Pascual-Leone himself and formula (1))
and information entropy (by formula (2)). To understand the message
of all this, we must explain in the following the theoretical
background of the so-called Erlangen school (Eysenck 1986, 1987) of
information psychology.
3 The Information Entropy of
Short-Term Memory Storage Capacity
Shannon`s (1948)
information entropy H is the logarithm of the number of microstates
consistent with our information. And Thom (1975; p. 157) stated: "To
reduce the information to this scalar measure (evaluated in bits of
accuracy gained) is to reduce the form to its topological
complexity." Each global state of a network can be assigned a single
number (Gibbs 1981), called the energy of that state, i.e. the
differences in the log probabilities, and hence information entropy
of 2 global states ("thoughts") is just their energy difference. By
extension of Shannon`s concept of channel capacity, already in 1959
Frank (see Lehrl and Fischer 1990) had claimed cognitive performance
to be limited by the channel capacity of short-term memory (Kyllonen
and Christal 1990). He argued that the capacity H of short-term
memory (measured in bits of information) is the product of the
processing speed S of information flow (in bits per second) and the
duration time D (in seconds) of information in short-term
memory. Hence
H (bits) = S (bits/s) x D (s) .
|
(3) |
As well known,
processing speed can be operationalised in test batteries by sets of
elementary cognitive tasks measuring choice reaction time or speed
of mental rotation, by scanning information in short-term memory, or
by measuring perceptual speed, by testing inspection time or time to
escape masking. Such elementary cognitive tasks permit the
measurement of individual differences while minimising variance
attributable to specific knowledge and acquired intellectual skills
and problem-solving strategies. Span and scan are only two sides of
the same coin: The greater the memory span, the faster the
processing speed. In appropriate samples both variables are
perfectly correlated (Cavanagh 1972; Lehrl et al. 1991). - see:
The Basic Period of Individual Mental Speed
(BIP)
4 Relationships between EEG Parameters and
Psychometric Results
In 1935, Gibbs et al. had already
documented that patients (sample size was 55) with petit mal
epilepsy show, in all cases during seizures, an outburst of spike
waves of great amplitude at a frequency of about 3/s. Now this
finding is part of our confirmed knowledge and can be read in every
textbook on EEG or epilepsy. From this in 1938, Liberson (see 1985)
had drawn the conclusion that all significant channels in EEG could
be n multiples of one fundamental frequency of about 3.3 Hz.
According to his empirical data the number of these multiples
(harmonics) is nine as the maximum of memory span (see Table 1).
Assuming these numbers one to nine to be quanta of action (as
Pascual-Leone 1970 did), we (Weiss 1986, 1987) obtain a relationship
between the classical formulae of quantum statistics and empirical
results of both EEG and psychometric research.
Table 3. Memory span
(corresponding to the number of an EEG harmonic), frequency of
EEG harmonics and mental speed and their relationships with
information entropy, power density of short-term memory
storage capacity, latencies of harmonics, and IQ (from Weiss,
1992b). |
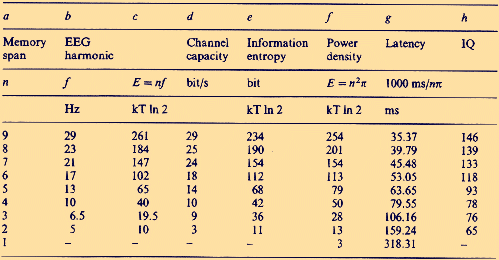 |
Column b: empirical
data from Liberson (1985). Column c: product of column b times
n. Columns a, d, e and h: empirical psychometric data from
Lehrl et al. (1991). Their sample size for standardizing the
test was 672 subjects. Notice that column e shows empirical
data and not the product of column d times n. Columns f and g
are purely theoretical. However, Liberson (1985) has published
similar empirical latency components of event related
potentials. |
In order to
find a theoretical frame for these connections (see Table 3), we
looked (Weiss 1986, 1989) for nearly all applications of wave theory
and found especially illuminating textbooks in such fields as
geophysics (for example Bath 1974) and optics (Yu 1976). Assuming
the numbers 1 to 9 to be harmonics, in accordance with Parseval`s
theorem (see Bath, 1974, or any textbook on communication theory)
the power spectral density E is given by the eigenstate
energy-frequency relationship (see Yu 1976)
where f is the
frequency. According to thermodynamics, the measurement of 1 bit of
information entropy requires a minimum energy of 1 kT x ln2 (Szilard
1929), where k is Boltzmann`s constant and T is absolute
temperature. (Of course, this cannot mean that the brain works with
this minimum of energy. The relationship, suggested by Table 3,
should hold for a macroscopic analogon, whatever it may be; compare
Haken 1988). During the duration of 1 perceptual moment 1 bit of
information is processed (Lehrl and Fischer 1988) per harmonic. That
means that 1 break of symmetry and 1 phase reversal after each
zero-crossing of a wave corresponds with a possible 1 bit decision
between two alternatives. Consequently, each degree of freedom of
oscillation corresponds to a macroscopic analogon of 1 kT, each
degree of freedom and translation (this refers to mathematical group
theory underlying quantum mechanics; compare Koenderink & Van
Doorn, 1990, and Goebel 1990) to an energy of kT/2 or its analogon,
respectively. Empirical analysis (Weiss et al. 1986) shows that
Liberson`s (1985) fundamental is lower than 3.3 Hz and in the range
between 3.1 and 3.3 Hz, i.e. near 3.14 Hz. Therefore, in the
following (as in Table 3) we will write for simplification np Hz. Because the frequency of harmonics
can be expressed as np Hz, for the
expected latencies of harmonics follows 1 000 ms/ np and for power density follows
E =
n²p (kT x
ln2).
The relationships in Table 1 are further supported by
data from Bennett (1972),who reanalysed the Ertl and Schafer (1969)
findings of a correlation between IQ and latencies of EEG evoked
potential components. Bennett (confirmed by Flinn et al. 1977)
accomplished a fourier transformation of the original data and found
that high IQ subjects (IQ above 123) go through 20 or more
perceptual moments per second, low IQ subjects (IQ below 75) only
through 8 moments or even less (compare Table 3, columns b and d).
This striking parallelism between EEG results and channel capacity,
measured with mental tests, is emphasised by results from Harwood
and Naylor (1969). Their subjects were confronted with stimuli of
defined information entropy (digits between 1 and 9 and numbers
between 1 and 32 presented single or in groups). 42 young university
students had a mean channel capacity of 21.4 bit/s; 105 "average
normal" adults who were 60-69 years old performed 14.2 bit/s; the
age group of 70-79 years (sample size was 67) achieved 12.9 bit/s;
and 13 subjects being 80 years and older 10.2 bit/s, thus reflecting
the usual decline of mental performance of old aged people. Pure
coincidence in this parallelism of channel capacity and EEG
frequencies (compare Table 1) seems impossible: neither Liberson nor
Lehrl, neither Bennett nor Naylor knew anything about the results
and theories of the others.
5 Discussion
The question whether interindivual variations in EEG
activity are related to IQ (Gasser et al. 1983) remained always
controversial. Because the probability amplitudes of the power
spectrum up to 30 Hz depend upon all the memory span of a given
individual, upon all its attentional resources, the power in a
selected frequency range (for example, in the alpha range) is no
good measure for a correlation with IQ. Consequently, the
probabilities Pt and Pe (see Table 2) should correspond to the
probability amplitudes of the evoked response of a given
individual.
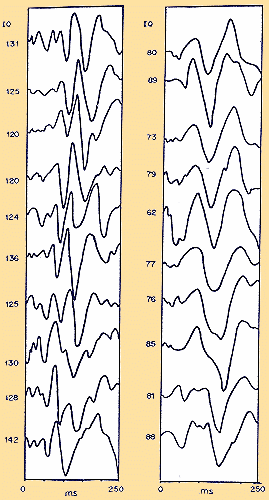 |
Fig.1 Evoked potential waveforms for ten high and ten low Iq
subjects (ertl and Schafer 1069). The individual IQ scores are
shown to the left of each waveform. (Note, that only the IQ of
individuals is given and not also their respective age. Ertl
and Schafer's random sample of 573 primary school purpils
comprised grades 2, 3, 4, 5 and 8; and younger children with a
given IQ have a lower memory span then older children of the
same IQ.)
| In
1969 Ertl and Schafer published data on a correlation between IQ and
latency components of evoked potentials. Hoewever, some authors
could (Perry et al. 1976; Hendrickson and Hendrickson 1980) and many
could not replicate their findings or only with substantially lower
correlations (for the ongoing discussion see Rothenberger and
Meyer-Dittrich 1984; Eysenck 1986; 1987; Weiss 1989). Why? Ertl and
Schafer numbered up to 250 ms the peaks and throughs from the left
(after stimulation) and they wrote: "The average evoked potentials
of the high IQ subjects are more complex, characterized by high
frequency components in the first 100 ms which are not observed in
the potentials of the low IQ subjects." However, by numbering the
peaks from the left, Ertl and Schafer (1969) measured in high IQ
subjects latencies of the ninth, seventh, and fifth harmonic. (And
the third peak, numbered E4 by Ertl and Schafer, had no real
counterpart at all in the waveform of low IQ subjects.) In low IQ
subjects positive peaks, caused by higher harmonics, cannot be
detected and peaks of the same poststimulus order (and before 250
ms) represent the fifth and third harmonic. (Because the
time-reversed fundamental 1000 ms/1p itself, the famous P3OO, is
lacking in the Ertl and Schafer data covering only the time range up
to 250 ms after stimulus.) However, replicable comparisons of
latencies can only be made on the basis of peaks or better
zero-crossings which are numbered from the P300 backwards (Haier et
al. 1984; Weiss 1989).
When we estimate in Fig. 1 the number
of zero-crossings (also denoted as nodes or nodal points) of the
waveform (an exact zero-volt-line was not given by Ertl and
Schafer), we can easily see that in high IQ subjects this number is
about 8, in low IQ subjects about 4 zero-crossings . According to
the Wentzel-Brillouin-Kramers (WBK) method of quantum mechanics
(Wentzel 1926), the number of zero-crossings (compare Fig. 2) is
identical with the quantum number. From this follows: The number of
zero-crossings of an evoked potential up to the P300 is identical
with memory span (i.e. with the number of macroscopic ordered
states).
In 1980, Hendrickson and Hendrickson confirmed the
correlation between IQ and evoked potential components using the
"string" measure. Because the higher IQ subjects have more complex
waveforms than the lower IQ subjects "an obvious measure was to
think of the waveform as a line. If the line were straigthened out,
the more complicated waveform would be longer, and the simple ones
would be shorter," for a time range up to the P300. However, is this
lenght of the waveform, Hendrickson and Hendrickson's "string"
measure, dependent upon something else than the power spectral
density of the evoked response?
The physical term power is
used because it is a measure of the ability of waves at frequency f
to do work. The power spectrum of the EEG describes the total
variance in the amplitudes due to the integer multiples of the
fundamental frequency. In order to calculate power density in this
way, the waveform must be squared and then integrated for the
duration of its impulse respone, i.e. the duration of the transient
of 1 complete wave packet. Theoretically, the energy E of the
impulse response and energy E, given in Table 3, must be identical.
What in an evoked response can be found, is not only the response to
a given stimulus, a given signal, but also a specific impulse
response dependent upon the metabolic mobilisation of brain energy
by a given individual. When a stimulus is fed into the brain, the
short-term memory wave packet immediately operates on this input,
and the result is the corresponding output signal ("a thought"). The
mathematical relationship is that the output is equal to the
convolution (time reversal) of the input with the short-term memory
impulse function (Crick et al. 1981).
Time reversal (the
concept of convolution in terms of communication theory) explains,
why the higher harmonics occur first after a stimulus. - Surveying
data from a very large number of psychophysiological experiments of
other authors or published by Geissler himself and coworkers,
Geissler (1987, 1990) drew the conclusion that the temporal
architecture of mental processes presupposes an universal constant K
of approximately 4.5 ms. His theory assumes that all conscious
information processing is based on integer multiples of K and chains
of multiples as 2K, 3K, 2², 4² and so on (compare also Stroud 1955,
who made a similar assumption, based on a secondary analysis of
empirical data by Von Bekesy). Multiples as 4, 8, 16, 20, 24, and 28
are preferred.
The Geissler-Stroud-theory has also its
counterpart in Table 3. The smallest possible power and time
difference, the difference K between the latencies of 9th (35.37 ms)
and 8th (39.79 ms) harmonic of the wave packet, is 4.42 ms, itself
the 1/72nd part of the fundamental. 9 K is the difference to the 8th
harmonic, 12K to the 6th, 18K to the 4th, 24K to the 3rd, 28K to the
2nd. Other important multiples as 3K, 4K, and 10K can easily be
identified as differences between harmonics. All higher multiples of
K, which are of importance in Geissler`s theory (1990), as 30, 32,
36, and 64 are differences to the 2nd harmonic and the fundamental,
respectively. And of course, these higher multiples are themselves
cascades of multiples.
What can tell us communication theory
on the meaning of such cascades? If ft(t) is a bandlimited signal
with a spectrum which is np Hz,
then it is determined by its values at the discrete set of points
equally spaced at m intervalls of K. Shannon`s sampling theorem (see
Jerri 1977) allows the replacement of any continuous signal f(t) by
a discrete sequence mK of its samples without the loss of any
information. If time divided into equal intervals K ms long and if 1
instantaneous sample is taken from each interval in any manner, then
a knowledge of the magnitude of each sample plus a knowledge of the
instant at which the sample is taken, contains all the information
of the signal. Sample and hold means to take the samples f(mK) from
the input signals at the time instants t = mK, and holds them
constant by delaying them until the next sampling. The probability
of the correct identification of a signal by a wave packet (or
wavelet in terms of geophysics, see Kronland-Martinet et al. 1987)
is a function of the probability of triggering a determined sequence
of frequencies and amplitudes. In other words, the empirical
background of the Geissler-Stroud-theory and the relationships of
this theory to the results in Table 3, all this is exactly that what
would be needed for perfect information processing in terms of
communication theory. - It is a pity, that the relationships betwen
zero crossings, eigenvalues, and spectral power density (for a
review see Robinson 1982), which are common knowledge in information
technology (Jerri 1977), are virtually unknown in cognitive
psychology and neurophysiology. Because it is impossible in the
context of such an article, to repeate a large number of formulae
and proofs, we must refer again to the above mentioned textbook
(Resnikoff 1989) as an obligatory background to understand our
following summarising: The most extreme compression of information
is represented by the eigenvalues (Kac 1966) of the spectrum. These
eigenvalues are always multiples of K. Their knowledge allows to
transmit and to reproduce any information. There are as much
eigenvalues of a spectrum as are harmonics (Wentzel 1926; see Fig.
2). Each eigenvalue of event related electrocortical potentials is
represented by a zero-crossing up to the P300 (Weiss
1989).
In view of the fact, that to the Soviet Livanov-school
of psychophysiology (see Lebedev 1990) the correlation between EEG
parameters and memory span (Polich et al. 1983) was well known for
at least a decade, it is surprising that this identity between
memory span and the number of zero- crossings has not been seen
earlier. That the number of zero-crossings of an epoch of the EEG up
to about 300 ms represents its power spectral density, was already
known to Saltzberg and Burch (see 1971) in 1959. However, these EEG
results were nearly forgotten before the advent of a new brand of
signal detection theory, stressing the importance of zero-crossings
(Niederjohn et al. 1987; Zeevi et al. 1987; Yuille and Poggio 1988).
Johnson (1988) stated that overall P300 amplitude represents
the summation of effects due to two independent variables:
subjective probability (P) and stimulus meaning (M). The amplitude
attributable to each of these two dimensions depends on the
proportion (T) of the overall stimulus information which was
transmitted to the subject. Thus:
P300 amplitude = f ( T x (1/P + M
))
|
(6) |
This attempt, to
explain the contribution of P300 to spectral density in terms of
information theory, has with our explanation in common that the
three dimensions (Johnson 1988) contain inherently the same
subjective (IQ) and objective (amount of information measured in
bits) elements as our approach, plus the information entropy of the
stimulus itself. We hope, that the intinuitive appeal of this
underlying congruency will be such a strong one that the two
approaches can be reconciled by future research into one formula and
one coherent theory of event-related potentials.
This article
tries to summarise well-established empirical facts and theoretical
conclusions and to avoid speculations. Therefore, it seems not
premature (compare also Basar 1988) to conclude: In analogy to
quantum mechanics the brain seems to be an ideal detector simply
measuring the energy of wave formes. No matter what the stimulus is
and how the brain behaves, the metric of signal and memory can
always be understood as a superposition of wave packets of np states of different energy and their
eigenvalues. Time is the currency (compare Jones 1976),
interconverting energy, information, and spatial distance (Weiss
1990).
References
Basar E (ed) (1988)
Dynamics of sensory and cognitive
processing by the brain Springer, Berlin
Bath M (1974)
Spectral analysis in geophysics.
Elsevier, Amsterdam
Bennett WF (1974)
The fourier transform of evoked
responses. Nature 239: 407-408
Cavanagh JP (1972)
Relation between the immediate memory
span and the memory search rate. Psychol Rev
79:525-530
Crick FHC, Marr DC, Poggio
T (1981).
An information-processing approach to
understanding the visual cortex. In: Schmitt FO, Worden EG, Edelman
G, Dennis C (eds.). The organization of the cerebral cortex. MIT
Press, Cambridge, pp 505-535
Ertl JP, Schafer EWP (1969)
Brain response correlate of
psychometric intelligence. Nature 223:421-422
Eysenck HJ (1986)
The theory of intelligence and the
psychophysiology of cognition. In: Sternberg RJ (ed) Advances in the
psychology of human intelligence. Vol. 3. Erlbaum, Hillsdale NJ, pp
1-34
Eysenck HJ (1987)
Speed of information processing,
reaction time, and the theory of intelligence. In: Vernon PA (ed).
Speed of information-processing and intelligence. Ablex, Norwood, pp
21-68
Flinn JM, Kirsch AD, Flinn
EA (1977)
Correlations between intelligence and
the frequency content of the evoked potential. Physiol Psychol
5:11-15
Fogleman G (1987)
Quantum strings. Am J Phys
55:330-336
Gasser T, Von Lucadou I,
Verleger R, Bächer P (1983)
Correlating EEG and IQ: a new look at
an old problem using computerized EEG parameters. Electroenceph Clin
Neurophysiol 55:493-504
Geissler H-G (1987)
The temporal architecture of central
information processing: evidence for a tentative time-quantum model.
Psychol Res 49:99-106
Geissler H-G (1990)
Foundations of quantized processing.
In: Geissler H-G (ed) Psychophysical explorations of mental
structures. Hogrefe and Huber, Toronto, pp 193-210
Gibbs FA, Davis H, Lennox
WG (1935)
The electroencephalogram in epilepsy
and in conditions of impaired consciousness. Arch Neurol Psychiat
(Chic) 34: 1133-1148
Gibbs WR (1981)
The eigenvalues of a deterministic
neural net. Math Biosc 57:19-34
Goebel PR (1990)
The mathematics of mental rotations.
J Math Psychol 34:435-444
Haken H (1988)
Information and self-organization: a
macroscopic approach to complex systems. Springer,
Berlin
Haier RJ, Robinson DL,
Braden W (1984)
Electrical potentials of the cerebral
cortex and psychometric intelligence. Person individ Diff
4:591-599
Harwood E, Naylor GFK
(1969)
Rates of information transfer in
elderly subjects. Austr J Psychol 21:127-136
Hendrickson DA, Hendrickson
AE (1980) The biological basis of individual differences in
intelligence. Person individ Diff 1:3-33
Jerri AJ (1977)
The Shannon sampling theorem - its
various extensions and applications: a tutorial review.Proc IEEE
65:1565-1596
Johnson R (1988)
The amplitude of the P300 component
of the event-related potential: review and synthesis. Adv
Psychophysiol 3:69-137
Jones MR (1976) Time, our
lost dimension: toward a new theory of perception, attention and
memory. Psychol Rev 83:323-355
Kac M (1966)
Can one hear the shape of a drum? Am
Math Monthly 73, part II:1-23
Koenderink JJ, Van Doorn AJ
(1990)
Receptive field families. Biol Cybern
63:291-297
Kronland-Martinet R, Morlet
J, Grossmann A (1987)
Analysis of sound patterns through
wavelet transforms. Internat J Pattern Recogn Artific Intell
1:273-302
Kyllonen PC, Christal RE (1990)
Reasoning ability is (little more than) working memory capacity.
Intelligence, 14, 389-433.
Lebedev AN (1990)
Cyclic neural codes of human memory
and some quantitative regularitities in experimental psychology. In:
Geissler H-G (ed) Psychophysical explorations of mental structures.
Hogrefe and Huber, Toronto, pp 303-310
Lehrl S, Fischer B (1988)
The basic parameters of human
information processing: their role in the determination of
intelligence. Person individ Diff 9:883-896
Lehrl S, Fischer B (1990)
Lehrl S, Gallwitz A, Blaha
L, Fischer B (1991)
Geistige Leistungsfähigkeit. Theorie
und Messung der biologischen Intelligenz mit dem Kurztest KAI.
Vless, Ebersberg
Liberson WT (1985)
Regional spectral analysis of EEG and
'active' and 'passive' intelligence. In : Giannitrapani D. The
electrophysiology of intellectual functions. Karger, Basel, pp
153-176
Miller GA (1956)
The magical number seven, plus or
minus two: some limits on our capacity for processing information.
Psychol Rev 63:81-97
Niederjohn RJ, Krutz MW,
Brown BM (1987)
An experimental investigation of the
perceptual effects of altering the zero-crossings of a speech
signal. IEEE Trans Acoust Speech Signal Processing 35:618-625
Pascual-Leone J (1970)
A mathematical model for the
transition in Piagetian developmental stages. Acta Psychol
32:301-345
Penrose R (1990)
The emperor` s new mind - concerning
computers, minds, and the laws of physics. Behav Brain Sci
13:643-706
Perry NW, Jr, McCoy JG,
Cunningham WR, Falgout JC, Street WJ (1976)
Multivariate visual evoked response
correlates of intelligence. Psychophysiol 13:323-329
Piaget J (1971)
The theory of stages in cognitive
development. In: Green ED (ed) Measurement and Piaget. Mc Graw
Hill, New York, pp 1-11
Polich J, Howard L, Starr A
(1983)
P300 latency correlates with digit
span. Psychophysiol 20:665-669
Resnikoff HL (1989)
The illusion of reality. Springer,
New York.
Robinson EA (1982)
A historical perspective of spectrum
estimation. Proc IEEE 70:885-907
Rothenberger A,
Meyer-Dittrich M (1984)
Beziehungen zwischen elektrischer
Hirnaktivität und Intelligenz im Kindesalter. Z Kinder-
Jugendpsychiatr 12:79-104
Saltzberg B, Burch NR
(1971)
Period analytic estimates of the
power spectrum: a simplified EEG domain procedure. Electroenceph
Clin Neurophysiol 30:568-570
Shannon CE (1948)
A mathematical theory of
communication. Bell System Technol J 27:379-432 and
623-656
Stroud JM (1988)
The fine structure of psychological
time. In: Quastler H. (ed) Information theory in psychology. Free
Press, Glencoe, pp 174-207
Szilard L (1929)
Über die Entropieverminderung in
einem thermodynamischen System bei Eingriff intelligenter Wesen. Z
Physik 53:840-856
Thom R (1975)
Structural stability and
morphogenesis. Benjamin Cummings, Reading, MA
Vichnevetsky R (1988)
Quantum properties of wave
propagation in periodic structures. Syst Anal Model Simul
5:103-129
Weiss V (1986)
From memory span and mental speed
toward the quantum mechanics of intelligence. Person individ Diff
7:737-749
Weiss V (1987)
The quantum mechanics of EEG-brain
dynamics and short-term memory. Biol Zentralblatt
106:401-408
Weiss V (1989)
From short-term memory capacity
toward the EEG resonance code. Person individ Diff
10:501-508
Weiss V (1990)
The spatial metric of brain
underlying the temporal metric of EEG and thought. Gegenbaurs
morphol Jahrb 136:79-87
Weiss V, Lehrl S, Frank HG
(1986)
Psychogenetik der Intelligenz.
Modernes Lernen, Dortmund.
Wentzel G. (1926)
Eine Verallgemeinerung der
Quantenbedingungen für die Zwecke der Wellenmechanik. Z Physik
38:518-529
Yu FTS (1976)
Optics and information theory. Wiley,
New York.
Yuille AL, Poggio TA (1988)
Scaling and fingerprint theorems for
zero crossings. Adv Comp Vision 2:47-78
Zeevi YY, Gavriely A,
Shamai S (1987)
Image representation by zero and
sine-wave crossings. J Opt Soc Am A 4:2045-2060
Home
The golden mean as clock cycle, the powers of the golden
mean and the golden mean shift as coding principle of the
brain
Harald
Weiss and Volkmar
Weiss
Preliminary
communication on an invited talk, held by Volkmar Weiss at the
5th Sommerfeld-Seminar 2002 of
the Arnold-Sommerfeld-Society, University
of Leipzig, Neuer Senatssaal, May 16th, 2002, 17
pm
At
first, Weiss recapitulated the empirical findings and theoretical
conclusions of two already published papers (see www.volkmar-weiss.de/publ10-e.html
and www.volkmar-weiss.de/publ9-e.html
, also
available on the server of Stanford
University, see http://www.slac.stanford.edu/~terryh/01Books/010Human-Paradigm/390Intelligence-IQ/030IQ-WM.html and http://www.slac.stanford.edu/~terryh/01Books/010Human-Paradigm/390Intelligence-IQ/020IQ-Brain-Energy.html
With
one decisive difference: Where you are reading Pi
(3,14) in these papers, you should read 2
Phi (3,236). (Without reading and understanding of these two papers,
the following new conclusions have no sense at all.) Some
colleagues complained that they could not open the above mentioned
URLs. I thought it would be already common knowledge: In order to
open such an URL, at first you have to write http://www.slac.stanford.edu/,
than add http://www.slac.stanford.edu/~terryh/
and click through the directory of Terryh in the order given above,
01Books/ followed by 010Human-Paradigm/ and so on or add it manually
step by step. Or try it with the support of http://www.google.com/ , filling
in the URL in the same manner, step by step.
We have the crucial question
to answer: Why is the clock cycle of the brain 2 Phi and not 1 Phi?
What is the advantage of the fundamental harmonic to be 2 Phi? Half
of the wavelength of 2 Phi , that means 1 Phi and its multiples are
exactly the points of resonance, corresponding to the eigenvalues
and zero-crossings of the wave packet (wavelet). With this property
the brain can use simultaneously the powers of the golden mean and
the Fibonacci word for coding and classifying. Compare, for
example, http://arxiv.org/pdf/cond-mat/9505072
and http://arxiv.org/pdf/cond-mat/9505072.
A binomial graph of a memory span n has n distinct eigenvalues and
these are powers of the golden mean. The number of closed walks of
length k in the binomial graph is equal to the nth power oft the
(k+1)-st Fibonacci number. The total number of closed walks of
length k within memory is the nth power of the kth Lucas number. See
Peter R. Christopher http://users.wpi.edu/~bservat/symsch97.html
An
extended publication, summarising the arguments in
favour of this new interpretation of the data - i.e. 2 Phi instead
of Pi - is in preparation. In order to accelerate the discussion, in
the following the most important links are given why Phi (the golden
mean, synonymously called the golden section, the golden ratio, or
the divine proportion), the integer powers of Phi, the golden
rectangle, and the infinite Fibonacci word 10110101101101 … (FW,
synonymously also called the golden string, the golden sequence, or
the rabbit sequence) are at the root of the information processing
capabilities of our brains.
Links:
Cited
from: http://home.earthlink.net/~sroof/Abraxas/sar/phi/phi.htm, Period Doubling Route to Chaos: It turns out when R = 2 Phi
= 2 times 1,1618 = 3,236 one gets a
super-stable period with two orbits. What this means is that Phi
enters into non-linear process as the rate parameter which produces
the first island of stability.
The
same holds for the Feigenbaum constants,
the length w1 is positioned at a = 2 Phi, see http://pauillac.inria.fr/algo/bsolve/constant/gold/gold.html . See also: bifurcation,
logistic parabola equation, edge of chaos. http://www.rootnode.com/Wiki/ProjectLab/LogisticMap
The
most important link is the Fibonacci Homepage. See especially the
four chapters Phi and the Rabbit sequence (= FW) and The rabbit
sequence (= FW) and the spectrum of Phi and Phi’s fascinating
figures (integer powers of Phi) and Integers as sums of powers of
Phi) http://www.mcs.surrey.ac.uk/Personal/R.Knott/Fibonacci/fibrab.html
http://www.geocities.com/CapeCanaveral/Launchpad/5577/musings/Attractors.html
The
following property of the FW is already applied in image processing
and recognition of handwriting:
The Phi
line Graph
If we draw the line y = Phi
x on a graph, (ie a line whose gradient is Phi) then we can see
the Fibonacci word directly.
Where the Phi
line crosses a horizontal grid line (y=1, y=2, etc) we write
1 by it on the line and where the Phi line crosses a vertical
grid line (x=1, x=2, etc) we record a 0.
Now as we
travel along the Phi line from the origin, we meet a sequence of 1s
and 0s - the Fibonacci sequence again!
1 0 1 1 0 1 0 1 1 0 1 1 0 1 0 1 1
0 1 0 1 1 0 1 1 0 1 0 1 1 0 1 ...
The
frequency of occurrence of either 1 or 0 is called the sampling
frequency by engineers.
Of fundamental importance: The Fibonacci word
and the spectrum of Phi
Let's look at the multiples of
Phi, concentrating on
the whole number part of the multiples of Phi. We will find
another extraordinary relationship. The "whole number part" of x
is written as floor(x) so we are looking at floor(i Phi) for
i=1,2,3,.. . In this section on the Fibonacci word will only be
interested in positive numbers, so the floor function is the
same as the trunc function.
The sequence of truncated
multiples of a real number R is called the spectrum of
R. |
Here are the first few numbers
of the spectrum of Phi, that is the values of the Beatty
sequence floor(Phi), floor(2 Phi), floor(3 Phi), floor(4 Phi),
..:- (Compare http://mathworld.wolfram.com/BeattySequence.html
)
i |
1 |
2 |
3 |
4 |
5 |
6 |
7 |
8 |
... |
i
Phi |
1.618 |
3.236 |
4.854 |
6.472 |
8.090 |
9.708 |
11.326 |
12.944 |
... |
trunc(i*Phi) |
1 |
3 |
4 |
6 |
8 |
9 |
11 |
12 |
... |
|
So the spectrum of Phi is
the infinite series of numbers beginning 1, 3, 4, 6, 8, 9, 11, 12,
... .
Now look at the
Fibonacci sequence and in particular at where the 1s occur:
I |
1 |
2 |
3 |
4 |
5 |
6 |
7 |
8 |
9 |
10 |
11 |
12 |
13 |
... |
Fibonacci
word |
1 |
0 |
1 |
1 |
0 |
1 |
0 |
1 |
1 |
0 |
1 |
1 |
0 |
... |
This pattern is
true in general and provides another way of defining the Fibonacci
word:
The 1s in the Fibonacci
word occur at positions given by the spectrum of
Phi and only at those positions. |
There is also a
remarkable relationship between the spectrum of a number and those
numbers missing from the spectrum.
Our brain uses for computing
inherent and inborn properties of the physical world. We have or learn into the
neural network of our brains the relationships between external
stimuli, the integer powers of the golden mean, the Fibonacci word
and Lucas numbers, the Beatty sequences of e, Pi, Phi and use
hundreds of similar relationships (many of them maybe still
undiscovered by contemporary mathematics) between numbers for
encoding and decoding simultaneously and unconsciously by wavelets.
Only a genius like Ramanajun had some access to this underlying
world of numbers. For example, he gave us: Phi (2) = 2 ln2, Phi (3)
= ln3, Phi(4) = 3/2 ln2, Phi(5) = 1/5 root of 5 lnPhi + ½ ln5,
Phi(6) = ½ ln3 + 2/3 ln2. See http://mathworld.wolfram.com/RamanujanFunction.html
Cited from: http://www.washingtonart.net/whealton/fibword.html A sub-word of the FW is any
fragment such as "abab" (or written 1010 as above) or "baa" (or
011). Certain patterns occur as observable sub-words of the FW "a,"
"b," "aa," "ab," "ba," etc., and certain conceivable patterns do
not.. At length one, two fragments are theoretically possible, "a,"
and "b." Both of them actually occur. At length two, the
theoretically possibilities are "aa," "ab," "ba," and "bb." Here,
the last one is never present, as we have seen. At length three,
only four of the eight possible patterns occur. They are "aab,"
"aba," "baa," and "bab." At length four, only five of the sixteen
possible patterns actually occur. At length five, only six out of
the thirty-two theoretically possible patterns are seen. In fact,
whatever the length of sub-word that is examined, it is always found
that the number of distinct sub-words actually occurring of that
length in the FW is always one more than the length itself. The
probability of finding a subword (and its parent or progeny, see the
following) of a wave packet with a maximum of up to nine harmonics
can be calculated by hidden Markov chains.
One pattern over another is the simple act of one pattern
generating another, as "abaab" generates "abaab" or even as sub-word
"bab" generates "aaba." At length 1, two legal sub-words are found,
"a" and "b." At length 2, three legal sub-words are found, "aa,"
"ab," and "ba." Here is where the new notion of descent comes in.
One can think of "aa" and "ab" as children of parent "a" because
both "aa" and "ab" can be created by appending a letter after the
pattern, "a". By the same logic, pattern "ba" has parent pattern,
"b." Continuing, one sees that "aa" is parent of "aab," that "ab" is
parent of "aba," and that "ba" is parent of both "baa" and "bab."
Simple arithmetic suggests that all but one of the sub-words of any
given length will act as parent for a single sub-word of length one
letter larger, while one sub-word alone will give birth to two
progeny. No other pattern is possible, for all sub-words must have
at least one child. Moving from length three to length four, we
note that "aab" produces "aaba," that "aba" gives rise to "abaa," as
well as to "abab, and that "baa" sires "baab," At the next level,
"aaba" produces "aabaa" and "aabab," "abaa" gives "abaab," "abab"
gives "ababa," and "baab" gives "baaba," and "baba" gives
"babaa."
It
turns out that the hyperparental sub-word, at any given length, is
precisely the FW itself of that length, written in reverse order.
That means that the FW reproduces itself upon reverse mapping (also
called block renaming or deflation in renormalization theories in
physics). This is the basic coding and search principle of
information in our brain. According with Zipf's law the most common
and short words have the highest probability of immediate access,
rare words a low probability. The coding itself needs learning. Only
the principle is the same, the details and content differ between
individuals.
The
best introduction into FW properties in this context you find in:
Schroeder, Manfred: Fractals, Chaos, Power Laws. New York: W. H.
Freeman 1991, pp. 45-57 and pp. 304ff.
The most comprehensive book for the physical implications
of the golden mean is: de Spinadel, Vera W.: From the Golden Mean to
Chaos. Vicente López 1998. (The book is still available, ISBN
950-43.9329-1)
Image processing, the FW as the reference pattern for
coding, see, for example, http://members.aol.com/kwpapke/GoldenDots.html
For
computer science the FW is no newcomer. Processing of strings of
symbols is the most fundamental and the most common form of computer
processing: every computer instruction is a string, and every piece
of data processed by these instructions is a string. Combinatorics
of words is the study of arrangement of such strings, and there are
literally thousands of combinatorial problems that arise in computer
science. You will find the relevant links and hundreds of papers and
books of the research front, if you feed into the searching robot of
Google: string, Sturmian, Fibonacci, combinatorics of words, code,
golden mean, string rewriting and combinations of these concepts.
See,
for example http://www.infres.enst.fr/~jsaka/PUB/FibGoldResume.html
Examples
of further links:
A
self-generating set and the golden mean http://www.research.att.com/~njas/sequences/JIS/VOL3/goldentext.html,
see also the One-Line Encyclopedia of
Integer Sequences A005614, A003849 by N.J.A.Sloane.
Scaling behavior
of entropy estimates, see http://www.geocities.com/ts271/schuermann-02.pdf
The
mathematical background behind al these relationships, of course, is
the prime number distribution, Ulam
spiral, Pascal's triangle and so on, for a summary of related links
see http://www.maths.ex.ac.uk/~mwatkins and http://www.maths.ex.ac.uk/~mwatkins/zeta/ulam.htm
.The most essential formulas are from Ramanujan where Pi, e and Phi are closed-form
expressions of infinite continued fractions, all three together
united in one such formula.
In
mathematics Cantorian fractal space-time
is now associated with reference to quantum systems. Recent studies
indicate a close association between number theory in mathematics,
chaotic orbits of excited quantum systems and the golden mean. See,
for example, http://xxx.lanl.gov/html/physics/0005067
and http://xxx.lanl.gov/abs/hep-th/0203086 and http://xxx.lanl.gov/abs/hep-th/0203086
for prime powers of the golden mean also http://www.liafa.jussieu.fr/~cf/publications/fibgold.ps , for the spatial entropy
two dimensional golden mean see http://www.math.nctu.edu.tw/People/e_faculty/jjuang.htm
Further
links are available, following http://mathworld.com/RabbitSequence.html and http://www.worldofnumbers.com/won118.htm
Prof.
Oleksiy Stakhov
has dedicated his life to the role of the golden section as a code,
see http://www.uem.mz/faculdades/ciencias/informat/docentes/stakhov/cont.htm
Fibonacci
L-sytems
http://www.math.okstate.edu/mathdept/dynamics/lecnotes/node59.html
Golden
mean frequency, golden mean scaling, period two orbit, renormalization, binary search tree,
Fibonacci shuffle tree, digital search tree, Feigenbaum-Shenker scaling constant, 2d Golden
mean or Hard square model, golden mean renormalisation of the Harper
equation, bit-string physics
The
golden mean in quantum geometry, knot theory and related topics http://www.ams.org/mathscinet-getitem?mr=2000c:11213
A
speculative paper by Nigel Reading, but hinting in the right
direction http://geocities.com/Area51/Starship/9201/phimega/phimega.html
Sturmian
sequences, entropy understood as pattern matching (see also the 1994
Shannon lecture by Aaron D. Wayner) http://math.washington.edu/~hillman/PUB/Fibonacci
and http://www-ext.crc.ca/fec/golden_Ref5.pdf
Optimal
search strategy of bees: a lognormal expanding spiral, based on the golden section, fits the
data best http://www.beesource.com/pov/wenner/az1991.htm.
This behaviour can be generalized to an optimal search strategy, for
example, for searching words in long-term memory (Zipf's law) or
filtering information from images. There are applications by Chaitin
and others.
It
is an astounding psychoacoustic fact, known as octave equivalence
that all known musical cultures consider a tone twice the frequency
of another to be, in some sense, the same tone as the other (only
higher). On the background of such observations Robert B. Glassman
wrote his review: "Hypothesized neural dynamics of working memory:
Several chunks might be marked simultaneously by harmonic
frequencies within an octave band of brain waves". See http://www.geocities.com/ripps/glassman.pdf Glassman's review is
essentially congruent with the papers by V. Weiss. We assume that behind octave
equivalence is the relation between 2 Phi and 1 Phi,
too.
As
you can see, the idea that the Fibonacci word can be understood or
can be used as a code, is not a new one.
There are already a lot of applications. However, new (by neglecting
a lot of nonsense with quasi-religious appeal) is the claim,
supported by proven empirical facts of psychology and
neurophysiology, that our brain uses the golden mean as the clock
cycle of thinking and hence the powers of the golden mean and the FW
as principle of coding.
In
1944 Oswald Avery discovered that DNA is the active principle of
inheritance. It lasted still decades before the genetic code was
known in detail and six decades later the human genome was decoded.
It will last some decades more, to understand the network of genetic
effects in its living environment. We believe that our discovery of
the fundamental harmonic of the clock cycle of the brain
can be compared with Avery's achievement.
In
1986 the senior author (V.W.) exchanged papers and letters with a
young man: Stephen Wolfram. One reason, why we do no present
a full paper now, is the expectation raised
by his book "A New Kind of Science". We are eager to read this book
before we write an extended new publication of our own. Today, May 17th,
the book is still not available in Germany.
The
authors: Harald Weiss
(Nuremberg), born in 1971, Dip. engineer of information technology;
PD Dr. Dr. habil. Volkmar Weiss (Leipzig), born in 1944.
|